Stay Up to Date
Submit your email address to receive the latest industry and Aerospace America news.
The Materials Technical Committee promotes interest, understanding and use of advanced materials in aerospace products where aerospace systems have a critical dependency on material weight, multifunctionality and life-cycle performance.
Material designs created by artificial intelligence and machine learning performed the same in models as those created through physics-based modeling, according to analysis performed between January and April by assistant professor Pinar Acar of Virginia Tech and her colleagues. The researchers applied the artificial intelligence/machine learning-based inverse design methodology to materials including titanium-aluminum alloy, from which parts of jet engines can be made including their compressor blades. In this methodology, designers begin with desired outcomes. If this method is adopted, location-specific properties of components will be optimized through the inverse design of underlying microstructural and processing parameters. The ability to optimize the macro-scale response by designing the microstructures and processes improve the performance and enable the integration of the material design into manufacturing.
In the field of bonded joints, research continued this year under a program created in 2020 called Thermoplastics Development for Exploration Applications, in which the bonding would be accomplished by applying heat to weld the composites together. This research could someday lead to the ability to create welded thermoplastic composite joints in space. Four candidate materials remained under consideration this year. Also, in June, virtual testing was performed on a digital composite lap joint through finite element analysis. This research into welded bonded joints builds on work with adhesively bonded joints that was conducted under NASA’s Composites Technologies for Exploration project, or CTE, which concluded in 2021. At the moment, incorporating adhesively bonded joints into a structure requires applying high knockdown factors, or KDFs, to the predicted strength of the bonds to ensure, for example, that a load will not exceed the actual strength of the bond. The material and process variability, widely varying geometric configurations and concentrated loads due to discontinuities motivate these KDFs in bonded joint designs. If these KDFs could be lowered, weight could be saved, something especially important in space launch applications. So, research was conducted with Space Launch System-scale structures under the agency-wide CTE project under the Game Changing Development program. A building-block approach was used to investigate the behavior and demonstrate the capabilities of longitudinal joints and circumferential end ring joints. The material used for the circumferential end ring was an advanced 3D-woven composite developed and manufactured by Bally Ribbon Mills and Cornerstone Research Group. The longitudinal joint and circumferential-joint test articles achieved greater than two times the design limit load, demonstrating the robustness of the bonded joint technology.
Turning to ultra-strong composites, researchers from the University of Utah this year contributed to the inverse approach by leveraging machine learning to reduce computational load in the design of these composites. In the field of carbon nanotubes, or CNTs, researchers working with funds from NASA’s Institute for Ultra-Strong Composites by Computational Design in February applied machine learning to devise a clever shortcut for determining global strength based on local measurements. Ashley Spear from the University of Utah and Gregory Odegard and Susanta Ghosh, researchers at Michigan Technological University, applied molecular dynamics simulations to estimate pullout strength — the force needed to drag a nanotube out of the matrix — as an indication of interfacial interactions between CNTs and the surrounding polymer. To avoid calculating every possible interaction, the researchers used representative values of interfacial strength in a CNT-epoxy system to train a machine learning algorithm to predict pullout forces. The model promises to allow exploration of the interface between CNTs and the polymer matrix, optimizing the chemical environment to maximize bulk-scale mechanical properties of CNT-polymer composites. The team is working on machine learning models that provide an even better picture of the connections and interactions between polymer chains on CNTs and in the matrix.
Contributors: Pinar Acar, Gregory Odegard and Evan J. Pineda
Stay Up to Date
Submit your email address to receive the latest industry and Aerospace America news.
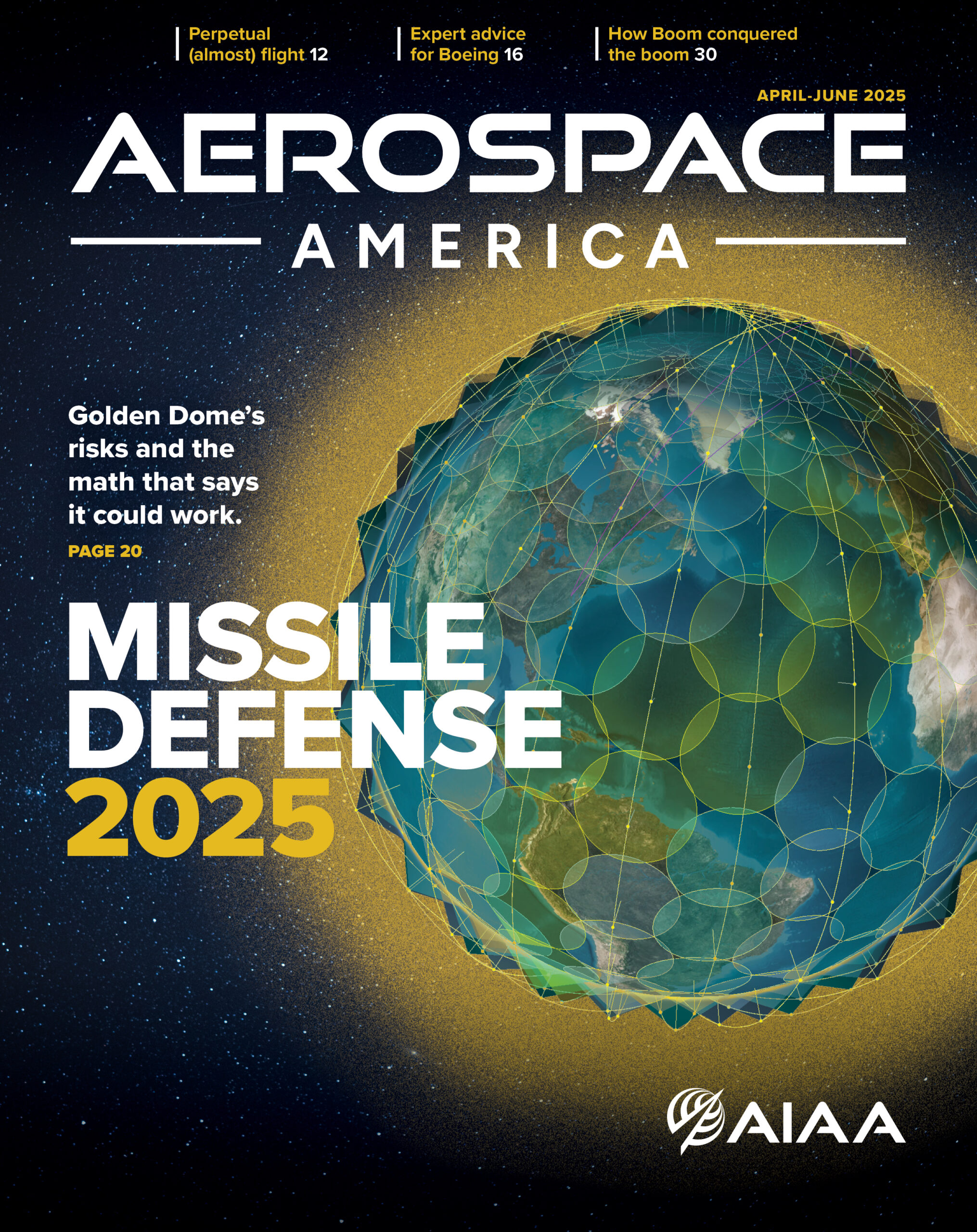