Seeing deep inside engines with X-rays
October 2020
Argonne National Laboratory outside Chicago is creating a research program that will empower investigators to apply the sensing qualities of X-rays to the challenge of seeing what goes on inside scramjets, rotating detonation engines and other hypersonic propulsion concepts. The program’s architects describe the technology’s heritage and its time- and cost-saving benefits.
BY BRANDON SFORZO, PRITHWISH KUNDU AND PINAKI PAL
A jet engine’s ability to lift us into the skies begins with a spray of fuel. The injected liquid joins with a stream of pressurized air and burns within the engine’s combustor, generating thrust — and you’re ready for takeoff.
Every engine, jet or otherwise, today benefits from the engineering community’s ever-growing understanding of this fuel injection process, a multiphase interaction so complex that it offers continual opportunities to tune performance. At the U.S. Department of Energy’s Argonne National Laboratory in Lemont, Illinois, we have a long history of studying this dynamic in automotive systems, by projecting powerful X-rays at spraying hardware and turning the resulting measurements into computational models.
Over the past year, we’ve begun to explore how these same techniques can be applied to the aerospace world. In partnership with industry, academia and other government agencies, we’re developing a unique research program that will help build not only the next generation of gas turbine engines, but emerging technologies, such as rotating detonation engines, to propel rockets and hypersonic jets.
X-ray vision for propulsion
Computer algorithms can help us capture and predict the intricacies of fuel sprays, but a model is only as good as the real-world data that shapes it. For that, we turned to Alan Kastengren, the physicist who oversees the 7-BM beamline, a pathway for X-ray light to be directed from the Advanced Photon Source, a ring-shaped particle accelerator at the Lemont campus. This beamline is one of approximately 60 specific pathways, each dedicated to a unique methodology for X-ray diagnostics, across a wide range of scientific disciplines, at the APS, one of DOE’s Office of Science User facilities. The 7-BM beamline is optimized for high-resolution fluid dynamics measurements, which means scientists and engineers can train a variety of X-ray sensitive detection equipment on fuel sprays and hardware to characterize what happens to the atomized liquid at the earliest phases of injection into the combustor.
The Vehicle Technologies Office in the DOE’s Office of Energy Efficiency and Renewable Energy funds the fuel spray research program relevant to gasoline and diesel direct injection.
Fuel spray studies at many other research institutions have relied on laser illumination to view liquid sprays prior to combustion, but visible light lasers are limited in a couple of ways. First, they cannot peer directly into the hardware at the point where fuel is being injected. Second, laser light tends to scatter when it hits many fuel droplets, much as sunlight is blocked by passing clouds. The extremely intense X-rays at the Advanced Photon Source don’t interact with the droplets the same way that laser light does. So, the beamline can help measure the distribution of fuel close to where it gets injected.
Stitching together thousands of time-resolved images, we can reconstruct across milliseconds how fuel droplets are formed and ripped apart, how they splash on the inner surface of the device, and where they move from there. In a study published in December by the American Society of Mechanical Engineers, Sforzo and four co-authors examined spray nozzle hardware and geometries provided by the National Jet Fuels Combustion Program, finding that the injected fuel behaved differently than expected based on previous characterization work.
This behavior hasn’t been studied very thoroughly until now. Armed with the novel data, computational fluid dynamics experts in industry and research community can construct much more robust and realistic modeling boundary conditions — the parameters within which a simulation will run. Boundary conditions set the context for a given gas turbine simulation: the pressure, velocity and temperature at which the fuel is injected, for example, or the cone angle of the spray, which has an effect on fuel distribution.
Furthermore, the quantitative validation data serves as a cross-check on the predictive performance of developed models. Taken together, all of these factors have a cascading effect on the performance of a jet engine, which is why computational modeling is critical in pinpointing the most efficient engine designs and fuels.
Predicting the future of fuel
One of the biggest computational challenges for gas turbine applications is that the size of each combustion simulation is significantly larger than any automotive one. Our numerical simulations generate terabytes of data. To analyze these terabytes, we turned to supercomputing resources such as the Theta system at the Argonne Leadership Computing Facility, another Office of Science user facility. In a 2018 study, our high-fidelity model compared two jet fuels with respect to lean blowout, in which the combustor’s flame extinguishes when the supply of fuel goes down.
This kind of predictive modeling saves engineers time and money by steering them toward the engine design parameters that are likely to work best, helping to avoid building a test engine that’s bound to send everyone back to the drawing board. But the computer simulations themselves involve a significant cost because they often run for months on a supercomputer.
To speed up the process, we are turning to a form of artificial intelligence known as deep learning. Deep neural networks “learn,” in a hidden manner, to be efficient representations of physical phenomena from voluminous data, which can improve the efficiency of predictive modeling. With these compact representations, we can turn terabytes of observational data into physics-informed surrogate models that run very quickly, instead of running thousands of computational fluid dynamics simulations. We’re using novel deep learning techniques in collaboration with the Raytheon Technologies Research Center of East Hartford, Connecticut, for example, to enable faster optimization of combustor and turbine cooling designs for modern aero-engines, while reducing the computational expense of fluid dynamics models.
A foundation for hypersonic flight
In the near term, the insights we gain from this novel combination of X-ray experiments and computational modeling can help engineers and designers maximize the efficiency and performance of next-generation gas turbine engines. And given that the combustor lies at the heart of any propulsion system, our work also applies to the longer-term vision of hypersonic flight and the development of advanced rotating detonation engines, RDEs, for rocket propulsion.
Unlike a conventional gas turbine engine, which combusts fuel at a constant pressure, an RDE confines a continuously rotating shock wave in a ring-shaped channel, creating elevated pressures that result in self-sustaining ignition of the fuel. This detonative mode of combustion could power engines that are smaller and lighter, but with a higher thrust than their current counterparts.
So far, the promise of RDEs far outstrips their viability; they are chaotic, dynamic systems in which the combustion phenomena are difficult to control. Working in collaboration with the Air Force Research Laboratory and Convergent Science Inc., our group has developed high-fidelity numerical modeling tools that can accurately predict the combustion characteristics of RDEs operating with practical hydrocarbon fuels in a computationally efficient manner and help researchers bring one closer to reality.
We also partnered with NASA’s Langley Research Center in Virginia to implement tabulated combustion models — accelerated using deep neural networks — to model hypersonic propulsion for aircraft, such as scramjets. By the completion of the project at year’s end, the algorithms we develop will be integrated with NASA’s in-house computational fluid dynamics code, VULCAN-CFD, supporting hypersonic propulsion device development.
At AIAA’s SciTech 2020 Forum, we convened the first meeting of an advisory committee with several technology experts and program managers from original equipment manufacturers and government agencies to provide guidance on the most meaningful research avenues going forward. We are designing a common testbed for our unique experiments, where we can examine these important physics under even more realistic conditions, which will in turn strengthen the computational models.
By building a bridge between the current expertise and capabilities for automotive fuel combustion and those for almost any aerospace application, we are laying the foundation for a new era of aerospace propulsion research. The most exciting developments in this effort are yet to come.
Related Topics
Modeling and SimulationBrandon Sforzo is a mechanical engineer at Argonne National Laboratory. He conducts fuel spray research for automotive and aerospace applications.
Prithwish Kundu is a research scientist at Argonne National Laboratory focusing on turbulent combustion modeling and its applications to propulsion.
Pinaki Pal is a research scientist at Argonne National Laboratory focusing on computational fluid dynamics, turbulent combustion modeling, machine learning, computational science and high-performance computing.
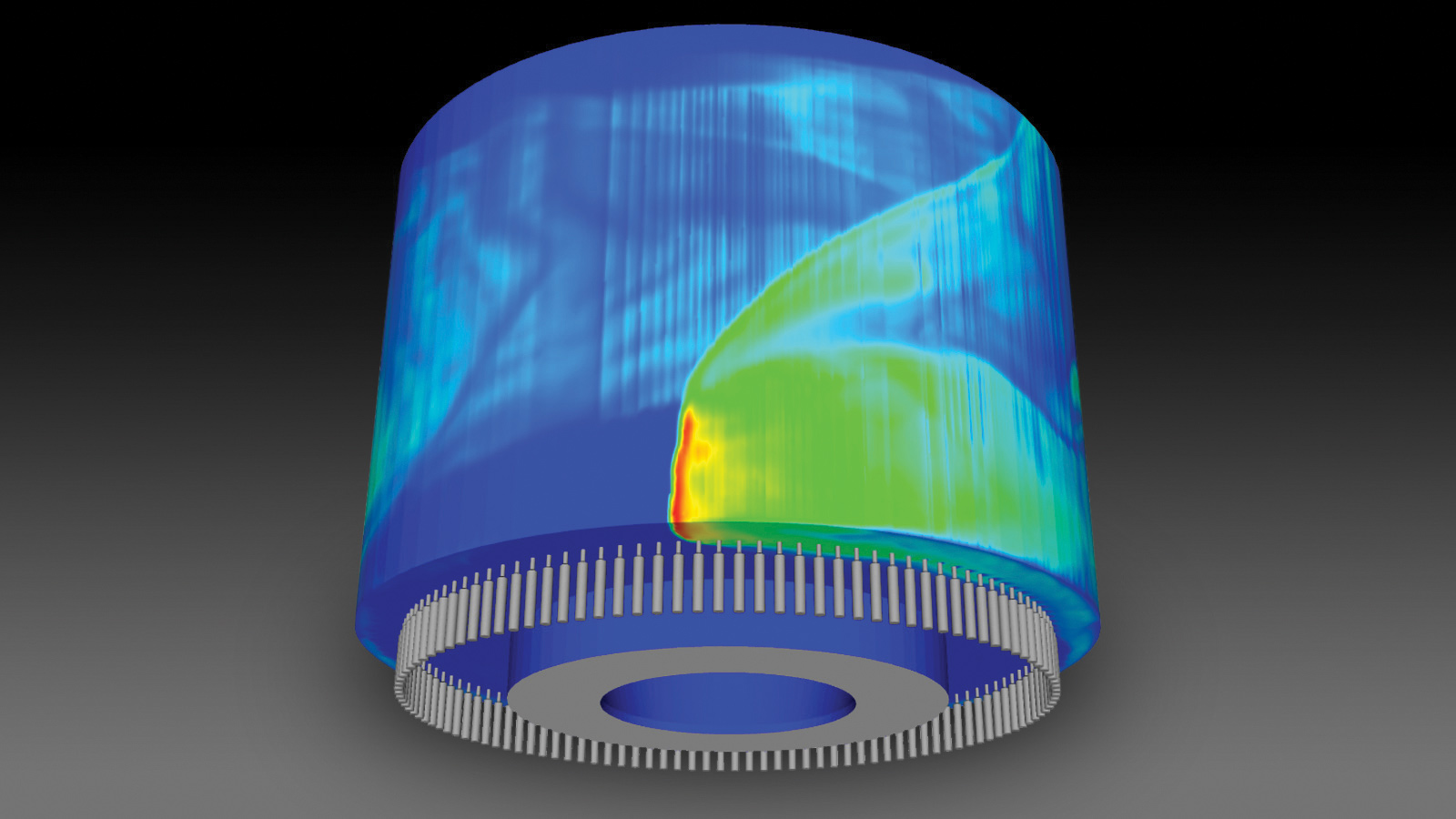
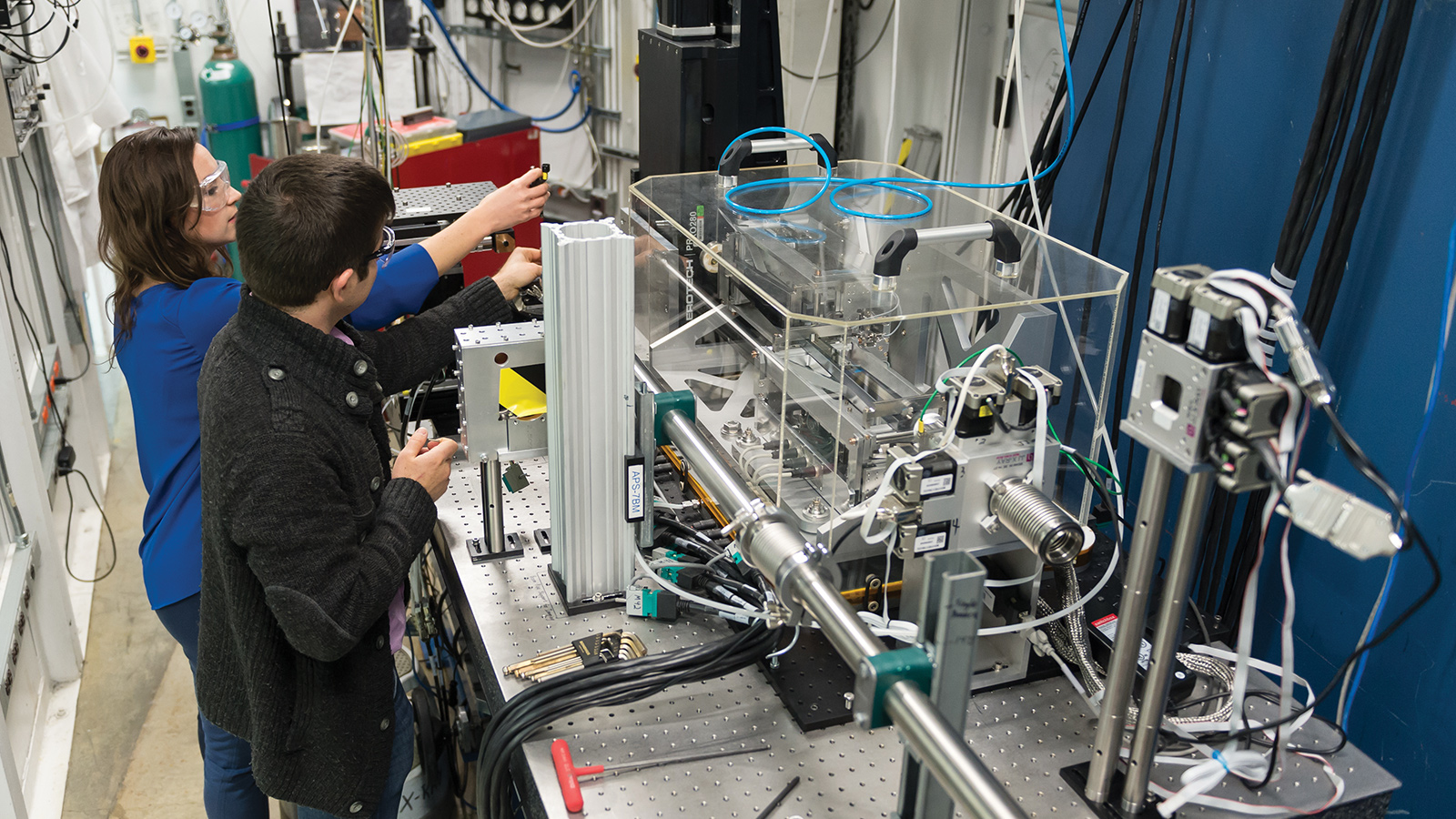