Stay Up to Date
Submit your email address to receive the latest industry and Aerospace America news.
The Fluid Dynamics Technical Committee focuses on the behaviors of liquids and gases in motion, and how those behaviors can be harnessed in aerospace systems.
Direct numerical simulations performed by researchers from Ohio State University, the National Institute of Aerospace in Virginia and NASA’s Langley Research Center in Virginia offered new insights into the problem of laminar-to-turbulent transition in hypersonic boundary layers in the presence of considerable nose bluntness. Between January and April, the researchers used DNSs of Mach 8 flow over a blunt cone in the axisymmetric nozzle of the Sandia National Laboratories’ hypersonic wind tunnel in New Mexico to mimic the disturbance evolution over a blunt cone in a virtual wind tunnel. The interplay between the freestream disturbances, the curved bow shock and the downstream entropy layer resulted in three-dimensional inclined structures that reflect the experimental schlieren findings above the boundary-layer edge. The DNS results also demonstrated that nonmodal optimal growth analysis is a useful technique for identifying the dominant components of the disturbance spectrum in a blunt cone boundary layer.
In March, SNL researchers performed high-fidelity large eddy simulations of turbulent flow over representative reentry vehicles over a range of angle-of-attack and flight speeds. They did this to understand the immensely harsh environment at hypersonic speeds resulting from severe, unsteady, turbulence-induced pressure fluctuations on the vehicle bodies, termed the random vibration environment. Often, researchers use reduced-order models derived from experimental data and high-fidelity computations to describe this environment, but these models are typically tuned to simplified configurations, such as a vehicle at a 0-degree angle-of-attack. This study will help expand the validity of a reduced-order model that characterizes the random vibration environment, thereby mitigating the use of current reduced-order models outside of their physically applicable scope.
At the AIAA Aviation forum in Chicago in June, researchers from Oklahoma State University, Virginia Tech, Emory University and Norwegian University of Science and Technology presented results of a hierarchical reduced-order modeling framework by integrating machine learning and variational multiscale approaches. This framework illustrates how to synthesize the strengths of physics-based modeling and machine learning algorithms. The main novelty of the proposed algorithm is that it builds reduced-order operators that are closest to the true interaction terms in the variational multiscale framework and leverages machine learning algorithms to reduce the projection error, further increasing the model accuracy. The numerical experiments for a two-dimensional vorticity transport problem showed that the proposed framework maintains the low computational cost of the base latent model while significantly increasing the model’s accuracy.
Experiments and simulations at North Carolina State University this year demonstrated how leveraging the latest advances in additive manufacturing with the flow diagnostics and simulation-guided experimental campaign can generate quick-turnaround scramjet inlet design tools and mature the technology readiness level to industry scale within a couple of years. Between January and July, researchers used pressure-sensitive paints to obtain two-dimensional maps of pressure, which leapfrogs the current measurements in the form of discrete pressure data along a line. They also leveraged additive manufacturing techniques for three-dimensional scramjet inlets. These techniques allowed for progress from a computer-aided design model to validation of quality datasets within a span of a few weeks. This collaborative effort helps answer how accurately an industry standard steady-state flow/turbulence model can determine the inlet performance at design and off-design conditions and what improvements can be made to the models to improve the accuracy of the calculations.
Contributors: Venkateswaran Narayanaswamy, Pedro Paredes, Omer San and Cory Stack
Stay Up to Date
Submit your email address to receive the latest industry and Aerospace America news.
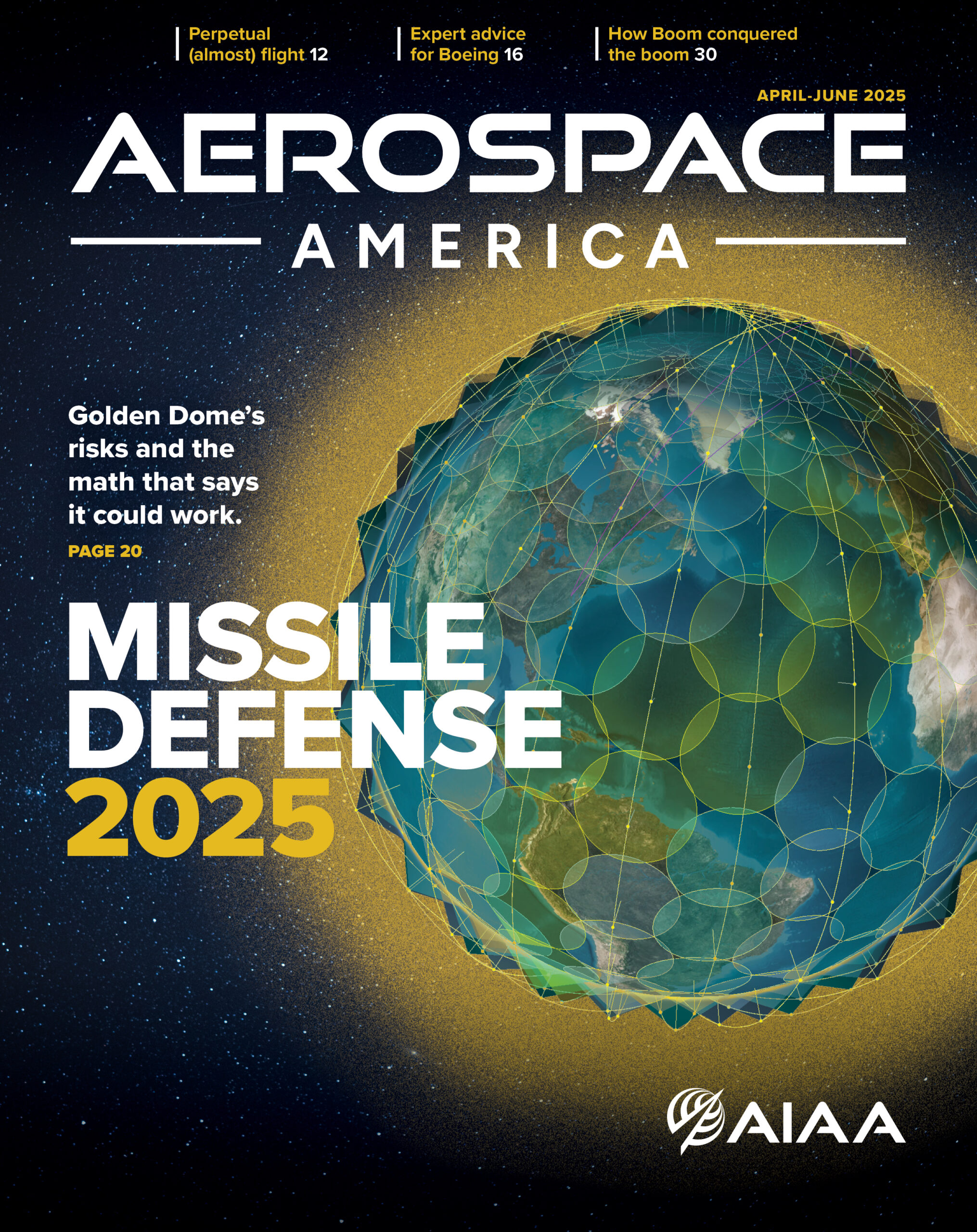