Stay Up to Date
Submit your email address to receive the latest industry and Aerospace America news.
Some people are genuinely scared that artificial intelligence will take over the world. Others believe AI is like magic and will solve all our problems. While the revolution does need to be managed through sound governance, what AI really amounts to is an emerging tool for augmenting our human capabilities.
Doing that effectively in the world of aerospace or anywhere else will require understanding the assumptions and limitations that underlie this technology.
Let’s begin with why we’d want to get help from machines in the first place, even if not necessarily AI. In general, we humans are interested in predicting the future, whether it’s the weather, stock values, shopping trends or developments in a host of other topics. In my line of work as a data scientist, I need to predict where two objects in orbit will be in the future. In order to know something, we must measure the attributes and behaviors of its constituent parts. Those measurements constitute data that might at first appear to be haphazard, but as more data is gathered, a skilled data scientist can find an emergent pattern or structure within the information. From that pattern, the scientist can develop a model that can be used to forecast what the next incoming data should look like.
The process to this point has always been labor intensive, involving algorithms but not AI. The difference between what we predicted and what we actually observe constitutes statistical surprisal. If we predicted exactly what we now observe, there is zero surprisal and thus nothing new to learn. Learning opportunities exist in the presence of surprisal, as we attempt to progressively minimize it. I first put this learning process into practice at the NASA-funded Jet Propulsion Laboratory to help me navigate a host of spacecraft to Mars, including the Mars Reconnaissance Orbiter. More recently, I’ve applied this process in my work to track anthropogenic space objects around Earth.
By now, at least some of us have tried the AI-driven Tesla autopilot and are aware that the Starlink satellites use AI to autonomously maneuver and avert potential collisions. While AI is far from ubiquitous, these examples illustrate the potential for AI to become a more widely applied helper — and perhaps a required one — for large-scale projects or tasks that must be undertaken frequently.
In terms of predictive analyses, AI is, in part, an attempt to accelerate the achievement of net-zero surprisal. However, an important caveat to all this is that at best, AI assumes that tomorrow will resemble today, drawing conclusions based on patterns and correlations observed in the past. This is referred to as training data, where the AI is taught what “truth” is and how it manifests. AI assumes the training data is complete, which in this context means that the data encompasses all the necessary information to describe what we’re interested in predicting. This training data is flawed when the model of today is limited, biased and incomplete, because it fails to sufficiently capture the full complexity and diversity of reality.
To consistently and confidently predict accurately and precisely, AI software needs a robust and diverse set of training data. Multiple models of possible todays are required to form a comprehensive understanding of what we wish to predict. Without a broad range of data that represents the possibility space of what we’re interested in, the predictions about tomorrow will be skewed and incomplete, unable to capture the nuances and complexities of the real world.
As a result of our incomplete understanding of the interdependencies and causal relationships within reality, the training data available for AI today is almost always incomplete. This incompleteness leads to inaccurate predictions, prescriptions or decisions, as the AI model lacks vital information required to capture the true essence of what we want to predict.
These limitations should serve as a reminder that this technology is a tool, not an omniscient entity. AI is only as good as the data it receives and the assumptions made. Garbage in, garbage out, as they say. It is essential to approach implementation of AI with a critical mindset and to understand the inherent biases and limitations that can influence its predictions.

About Moriba Jah
Moriba is a professor at the University of Texas at Austin and chief scientist at Privateer. He helped navigate spacecraft at NASA’s Jet Propulsion Lab and researched space situational awareness at the U.S. Air Force Research Laboratory, and is an AIAA fellow.
Related Posts
Stay Up to Date
Submit your email address to receive the latest industry and Aerospace America news.
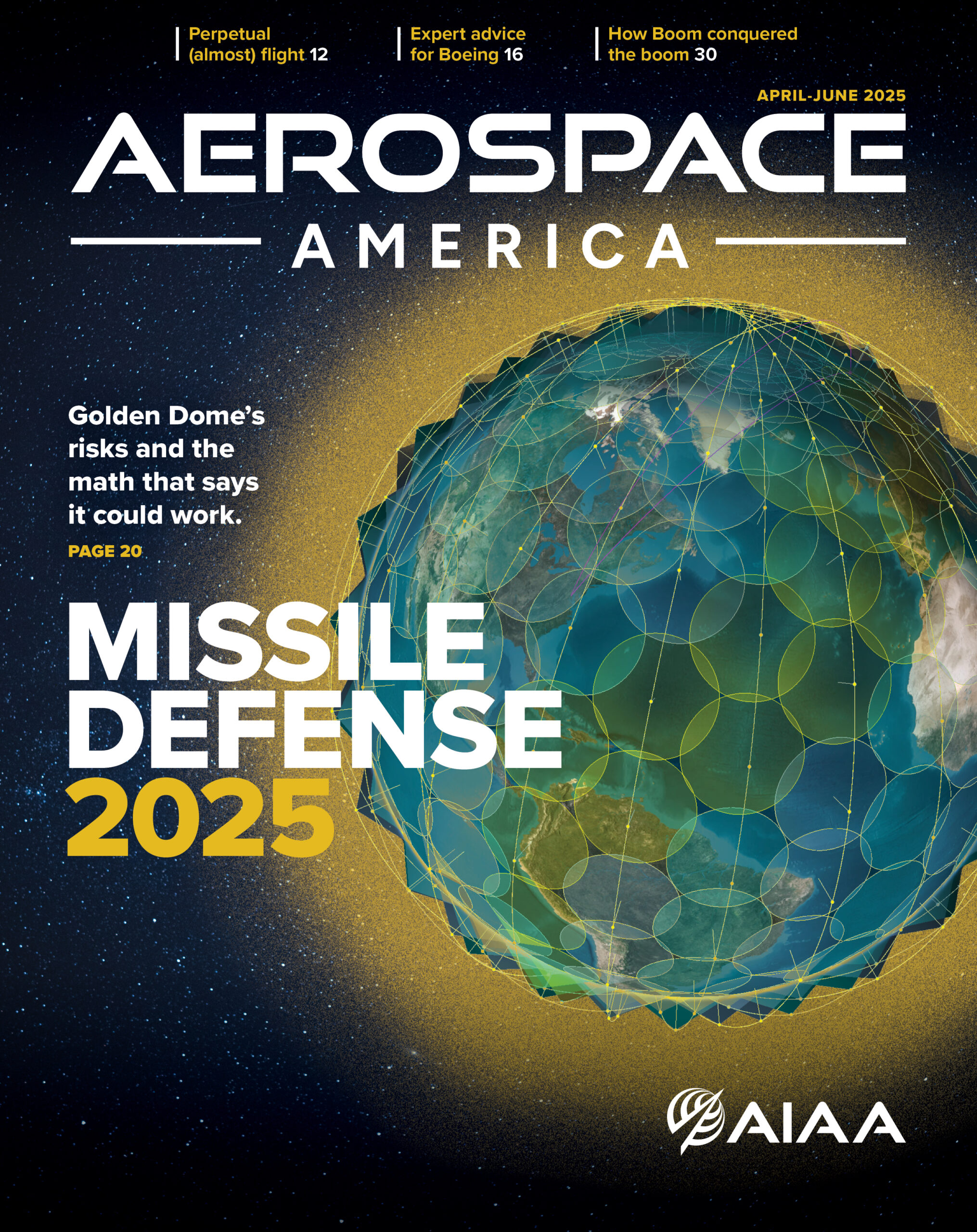